Product request
You are looking for a solution:
Select an option, and we will develop the best offer
for you
Your Wish Is My Command
![]() | Thirty-one years ago, a TV that responded to voice commands in the movie Back to the Future, Part II was an idea as fantastic as a flying car, almost everyone has access to devices with voice interfaces. In this article, we’ll explain how speech recognition works and show you how voice assistants operate, using Google Assistant as an example. |
Early experiments in voice recognition
![]() | Surprisingly, the first home product with a voice interface (VI) was a toy. In 1987, the Julie doll, which could be trained to recognise children’s speech, was released. Julie reacted to stimuli like turning off the light and read the books that came with it aloud. |
A few years later, the first voice-recognition dictation software appeared, in addition to devices for the visually impaired and people who couldn’t use computer keyboards due to physical limitations.
In 1990, a license for DragonDictate, the first ‘voice typewriter’, cost $9000.
Later in the 1990s, other voice interfaces were introduced to automate business processes. For example, the VAL portal from BellSouth processed telephone inquiries and told customers about the company’s services. Unfortunately, these early solutions were inaccurate and required lengthy training.
| The technology steadily improved over time and today, many of the ‘smart’ devices available to consumers come equipped with voice interfaces. Manufacturers of technology products have incorporated voice recognition into their devices to offer their customers greater ease of use and hands-free operation. Speaking is easier than typing when you’re in the midst of daily activities – while you’re driving or commuting, or when you’re in front of your TV. |
Present-day speech recognition systems help us find information, transcribe text, and schedule appointments. They are used in interactive self-service systems, for example, technical support services.
How does speech recognition work?
Devices perceive speech differently than humans. Instead of isolated words, a device hears a continuous signal, in which sounds flow smoothly into each other. A device will detect a single phrase pronounced with different intonations or voiced by different speakers as different signals. Because of the high degree of variability in human speech, voice recognition accuracy has not yet reached 100%. The main task of voice-recognition algorithms is to interpret what has been said regardless of the pronunciation peculiarities of the speaker or the presence of background noise and other interference. | ![]() |
Elements of a speech-recognition system
Speech recognition systems consist of four components:
- a sound-cleaning module (to remove background noise)
- an acoustic model (to discern the speech sounds)
- a language pattern (to predict the most likely word sequences)
- a decoder (to combine the data output from the acoustic model with the language pattern to provide the final result)
At each stage, the acoustic signal goes through a series of transformations. You can read more about these below.
1. Sound cleaning
The first task of a speech-recognition system is to evaluate the quality of the sound input and separate the desired signal from sound interference, or noise. Depending on the nature of the unwanted sound, different approaches can be used to filter speech from background noise.
Noise suppression
There are several ways to suppress noise in a speech-recognition system. One of them is to introduce artificial noise, including recordings of common man-made noises (for example, the sound of a car engine, wind, or rain), to the system to ‘teach’ the acoustic model to distinguish sound from background noise. However, when the system later encounters unfamiliar noise, the model will likely send an ‘error’ message.
Other noise suppression approaches rely on hardware solutions. Some smartphones are equipped with two microphones: the first microphone, located on the front of the device, catches speech with interference while a second microphone on the back picks up ambient background noise. In theory, all you have to do to get a clear signal is simply subtract the second sound signal from the first.
Extraneous voices
It is more difficult to single out a particular voice when several people are speaking at the same time than to distinguish speech from non-speech sounds. To isolate an individual speaker from other voices, the acoustic model adapts to the user’s voice and remembers the peculiarities of his/her pronunciation.
2. Dividing a speech signal into words
The next task for a voice-recognition system is to single out individual words in a continuous sonic flow and determine their meaning.
At its most basic level, speech can be seen as alternating sounds and silences. The silences can be understood as word ‘separators’.
To analyze a speech signal in terms of words and word separators, an audio recording is first split into frames, i.e., small sections that are approximately 10 ms in length. These frames are not strictly consecutive: the end of one section is superimposed on the beginning of another.
To determine which of the frames contain human voices, the system sets a limit. Values above the limit are considered to be words, while values below are understood as silence. There are several options for setting the limit value:
- Setting it as a constant (this constant can be used when sound is generated in the same way and under the same conditions).
- Defining a number of values that correspond to silence (if silence occupies a significant part of the recording).
- Performing an entropy analysis (this requires determining how strongly the signal ‘oscillates’ within a given frame. The amplitude of oscillations for silent parts of a recording is usually lower).
Of the three, entropy analysis is considered the most reliable, although it has its flaws. For example, entropy may decrease when vowels are drawled or increase with a slight noise. To resolve the problem, two concepts a ‘minimum distance between words’ and a ‘minimum word length’, are introduced. The algorithm merges fragments that are too short and cuts off noise.
3. Interpretation of words
![]() | Most often, neural networks, combined with an apparatus containing hidden Markov models, are used to interpret words. |
Hidden Markov models While researching early 20th century literary texts, mathematician Andrey Markov initially assumed the probability of the occurrence of a letter depended on the letter that preceded it. It turned out that this value remained constant in different parts of the same text. | ![]() |
Probability indicators are unique for each author. This makes it possible to use Markov models to spot plagiarism.
In Markov models, printed text consists of sequences of characters while speech is treated as a sequence of phonemes. While all of the symbols in written text are known, voice recordings contain the manifestation of phonemes and not the phonemes themselves (for example, there are several ways to pronounce the ‘R’ sound).
The device does not know which phoneme has been pronounced; it perceives only the parameters of the sound wave at a certain moment in time. In addition to estimating the probability of the occurrence of a particular phoneme, the system has to associate phonemes with appropriate signal variants.
Feedforward neural networks
Until recently, self-learning neural networks with numerous layers were most often used in speech recognition.
- These networks process information in one direction only, namely from input neurons to the output neurons.
- Several layers of neurons are hierarchically located between the input and the output, where the parameters of a higher level follow from the parameters of a lower level.
- Self-learning or unsupervised learning implies that the neural network learns to solve problems without outside intervention. The approach reveals hidden patterns between the objects of the training sample.
The result, representing a set of probabilities of the occurrence of a particular phoneme, is compared with the prediction of the Markov model. A pronounced sound is determined quite accurately.
Recurrent neural networks
Speech-recognition systems are gradually moving away from the use of simplified hidden Markov models. More and more, acoustic models are being built on recurrent neural networks, where internal memory and backpropagation are used for more efficient recognition.
Neurons not only receive information from the previous layer but also send the results of their own processing to themselves. This makes it possible to consider the order of data occurrence.
4. Phrasing
The principle of distinguishing phrases and sentences is very similar to word decoding. Previously, N-gram type models were used for this task, where the probability of occurrence of a word depending on N previous words (usu. N = 3) was determined based on the analysis of large text blocks. | ![]() |
Deep learning and development of recurrent neural networks significantly improved the linguistic model and allowed it to take into account the context of what was said. The restriction on the use of only N previous words also disappeared.
Linguistic models were now able to guess the words missed or not recognised for a number of reasons. This turned out to be especially important for languages with random word order like Russian, where not only the previous words were important but the entire phrase.
This is how most speech-recognition systems work. But it is not enough to understand what was said. To be useful, the system must also be able to respond to incoming commands: it needs to answer questions, open apps, and manage other functions. Voice assistants are responsible for these tasks.
Speech recognition in MAG425A
![]() | MAG425A is equipped with a voice-controlled remote and Google Assistant. The voice interface provides a completely new user experience. The main functions the voice assistant performs include
|
What is Google Assistant?
Google Assistant is a virtual voice assistant first introduced at the
Google Assistant uses computer-assisted learning and Natural Language Processing (NLP) technology. The system is able to single out sounds, words, and ideas in speech.
The Assistant operates a billion devices and supports over thirty languages, but the Android TV version only speaks twelve languages so far: English, French, German, Hindi, Indonesian, Italian, Japanese, Korean, Portuguese, Spanish, Swedish, and Vietnamese.
How does Google Assistant work?
First, the application records the speech it detects. Interpreting speech requires extensive computational power, so Google Assistant sends requests to Google data centres. When the sound data reaches on of these centres, the solid signal is divided into sounds. Google Assistant’s algorithm searches a database of speech sounds and determines which words are the best match for the recorded combination of sounds.
The system then singles out the ‘main’ words from the user’s statement and decides how to respond. For instance, if Google Assistant notices words like ‘weather’ and ‘today’, it will respond with today’s weather forecast.
![]() | Google servers send the information back to the device, and the Google Assistant app performs the desired action or responds with a voice. |
Google is changing the way Google Assistant works so that speech is recognised and commands are processed directly on the user’s device. Using the capabilities of recurrent neural networks, the company has developed a new model for speech recognition and understanding. The size of the database of acoustic models has undergone a hundredfold reduction, so the artificial intelligence of Assistant can already work locally. The application processes speech in real-time and with almost zero delay, even without Internet access. | ![]() |
The new-generation Google Assistant responds to a request almost ten times faster. Since 2019, Google’s new Pixel smartphone models have supported Google Assistant. In the future, the app will be available on other devices as well.
Today, Android TV’s voice interface is available not only to companies with million dollar budgets, but also to local IPTV/OTT operators. This is a great opportunity for operators to attract new audiences, create content searches and accessing services easier and more convenient for users so they can stand out against their competitors.
*Google and Android TV are trademarks of Google LLC.
Recommended
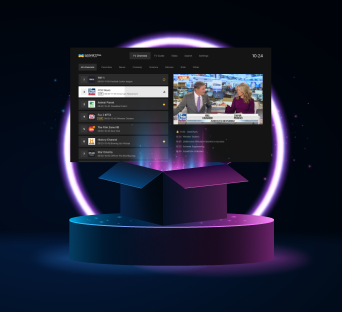
Ministra PRO without investments: how can an operator get new cost-free middleware?
Launching an IPTV/OTT project requires an operator to invest in market research, equipment, content purchase, advertising, user devices and many other investments. However, Infomir offers a cost-effective solution that allows operators to save money on one of the most important service elements by offering no-cost IPTV middleware.
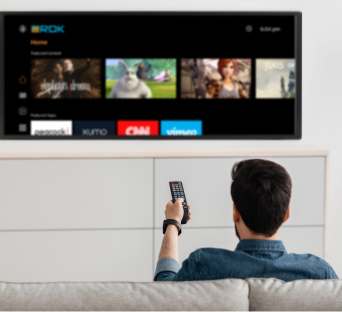
Future-proofing IPTV with RDK: convenient platform for operators
Every year, telecommunication technologies reach a new level, offering operators effective solutions and TV advancements. In this article we will focus on one of these advancements – RDK technology. We will explain why hundreds of operators choose this solution and why it has become so important to them.
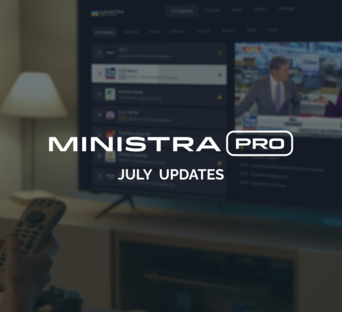
Ministra PRO: July updates
Vacation time is in full swing, and with members of the Ministra PRO team also getting ready to take their holidays, the July digest will be short. For this edition, we’ve gathered together all the major updates for this year so you can browse through them (while hopefully enjoying your own well-earned break!).